Steel corrosion is one of the most costly and pervasive challenges in infrastructure and construction. From bridges and pipelines to ships and skyscrapers, the gradual breakdown of metal structures can lead to massive financial losses, costing between 3-4% of the global GDP annually.
To tackle this issue, researchers at Lawrence Livermore National Laboratory (LLNL) have developed a breakthrough corrosion modeling framework that could significantly improve how engineers predict corrosion and design more durable materials. Recently published in Nature Communications, this work offers a new approach to predicting corrosion before it happens – a development that could help prevent material failure and extend the lifespan of critical infrastructure.
Why Steel Corrosion Modeling Matters
Corrosion often starts where it’s least expected – in the hidden, high-stress zones of a project. Until now, most strategies for corrosion prevention have relied on historical data from familiar alloys and well-documented environmental conditions. However, with evolving materials and increasingly aggressive environments, these legacy models are no longer enough.
LLNL’s researchers used advanced kinetic modeling to simulate corrosion at the nanoscale, revealing how protective oxide layers form, break down, and reform over time. These thin films are essential – if they dissolve or become permeable, corrosion sets in fast.
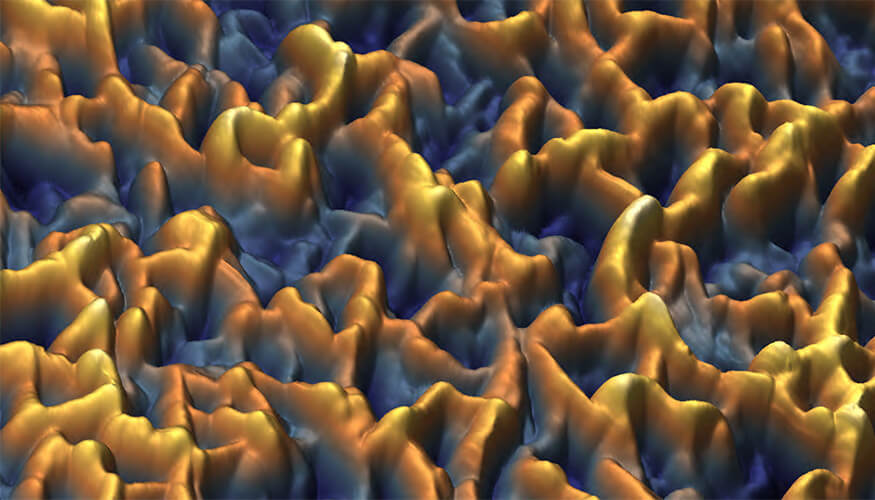
A Machine Learning Approach to Predicting Corrosion
To make their framework practical and scalable, the team integrated a machine learning-inspired model that can predict corrosion onset based on environmental and material factors – including pH, voltage, and composition. Instead of relying on trial and error, engineers could one day input these parameters into a digital tool and receive accurate predictions on corrosion risk.
This is a major step forward in material durability analysis, particularly for projects that involve novel alloys, complex material interactions, or highly variable environmental exposure.
The Mystery of the Intermediate Voltage Zone – Solved
One of the most valuable discoveries from this research was an explanation for what happens in the intermediate voltage regime – an area that has long puzzled engineers.
LLNL found that two processes – dissolution and reprecipitation – compete in this regime. As molecules leave the metal surface, mix with the environment, and redeposit, they reshape the oxide layer in unpredictable ways. The result is a protective barrier that may look stable but has completely different properties.
Even in the absence of applied voltage, placing dissimilar metals in close contact can create a kind of microbattery that triggers these same conditions. This has major implications for bridge design, shipbuilding, and other fields where mixed-metal structures are common.
Designing Corrosion-Resistant Infrastructure
By simulating corrosion processes in detail, this research opens the door to proactive material design. Rather than waiting for failure or relying on conservative safety margins, engineers will be able to use predictive models to inform their material choices and structural designs from the outset.
For anyone working on infrastructure durability, asset lifecycle planning, or corrosion engineering, this model will offer a smarter, more data-driven way to reduce risk and extend the life of built assets.
LLNL’s work is a great example of how data-driven modeling and machine learning can unlock new frontiers in material durability – and why paying attention to corrosion today can lead to stronger, smarter infrastructure tomorrow.